LIMITED TIME OFFER
Replace all of these

with a single tool for just $49 per month for your entire team
UNLIMITED USERS
UNLIMITED PROJECTS
UNLIMITED CHATS
UNLIMITED DOCS
UNLIMITED STORAGE
AND MORE..
Successfully Implementing a Predictive Maintenance Project
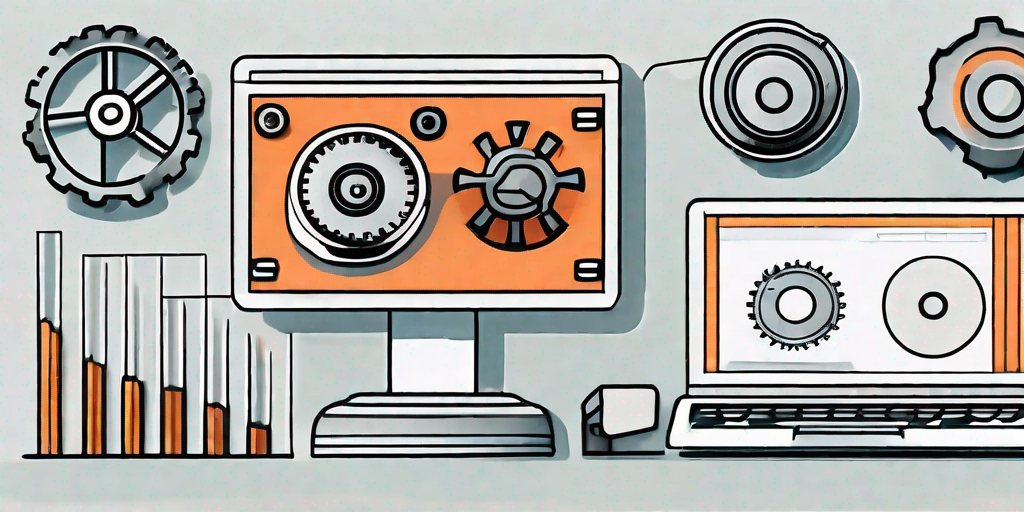
Predictive maintenance is a valuable strategy that can help businesses optimize their operations and reduce downtime. By utilizing advanced technologies and data analysis techniques, companies can proactively identify potential equipment failures and take preventive actions before they happen. However, implementing a predictive maintenance project requires careful planning, the right tools and technologies, and addressing various challenges along the way. In this article, we will explore the key components of a predictive maintenance project and the necessary steps to successfully implement it.
Understanding Predictive Maintenance
Before diving into the implementation process, it is crucial to have a clear understanding of predictive maintenance. Predictive maintenance involves using real-time data and analytics to predict when equipment failure is likely to occur and taking proactive measures to prevent it. Unlike traditional maintenance approaches that rely on scheduled inspections and routine replacements, predictive maintenance relies on data-driven insights to optimize maintenance activities.
Defining Predictive Maintenance
Predictive maintenance is a proactive maintenance strategy that uses various techniques, such as condition monitoring and data analysis, to predict equipment failures before they happen. By monitoring the health and performance of equipment in real-time, organizations can identify potential issues and take corrective actions to prevent costly breakdowns.
Condition monitoring is a key component of predictive maintenance. It involves the continuous monitoring of equipment parameters, such as temperature, vibration, and pressure, to detect any deviations from normal operating conditions. This data is then analyzed using advanced algorithms and machine learning techniques to identify patterns and anomalies that may indicate impending failures.
Data analysis plays a crucial role in predictive maintenance. By analyzing historical data and real-time sensor data, organizations can develop predictive models that can accurately forecast equipment failures. These models take into account various factors, such as equipment age, usage patterns, and environmental conditions, to provide accurate predictions.
The Importance of Predictive Maintenance
Predictive maintenance offers several benefits for businesses. First and foremost, it helps prevent unplanned downtime, which can have significant financial implications. By identifying issues early and scheduling maintenance activities accordingly, businesses can avoid costly production disruptions and minimize revenue losses.
Unplanned downtime not only leads to lost production time but also increases the risk of secondary damages. When equipment fails unexpectedly, it can cause collateral damage to other components or systems, resulting in additional repair and replacement costs. Predictive maintenance helps mitigate this risk by enabling proactive maintenance actions to prevent failures before they occur.
In addition to reducing downtime, predictive maintenance also helps optimize maintenance costs. By focusing maintenance efforts on equipment that truly needs attention, organizations can avoid unnecessary maintenance activities and allocate resources more efficiently. This can result in significant cost savings in the long run.
Furthermore, predictive maintenance enables organizations to extend the lifespan of their equipment. By identifying and addressing potential issues early on, organizations can prevent premature equipment failures and maximize the lifespan of their assets. This not only reduces capital expenditures but also improves overall operational efficiency.
Another advantage of predictive maintenance is improved safety. By proactively addressing equipment issues, organizations can reduce the risk of accidents and injuries caused by equipment failures. This not only protects the well-being of employees but also helps organizations comply with safety regulations and standards.
Lastly, predictive maintenance allows organizations to shift from reactive to proactive maintenance strategies. Instead of waiting for equipment failures to occur and then reacting to them, organizations can take preemptive actions to prevent failures from happening in the first place. This shift in approach can lead to a more efficient and reliable maintenance program.
Key Components of a Predictive Maintenance Project
Implementing a predictive maintenance project involves several key components that are essential for its success. Let’s explore these components in detail.
Predictive maintenance is a proactive approach to equipment maintenance that aims to predict and prevent failures before they occur. By utilizing advanced technologies and data analytics, organizations can optimize maintenance schedules, reduce downtime, and extend the lifespan of their equipment.
Necessary Tools and Technologies
One of the critical components of a predictive maintenance project is selecting the right tools and technologies. This includes equipment sensors for data collection, data storage and processing solutions, and analytical tools for predictive modeling and visualization.
When choosing equipment sensors, organizations must consider factors such as accuracy, reliability, and compatibility with the equipment being monitored. For example, in the case of rotating machinery, vibration sensors can provide valuable insights into the health and condition of the equipment.
Data storage and processing solutions are crucial for handling the vast amount of data generated by the sensors. Cloud-based platforms and big data technologies offer scalability and flexibility in managing and analyzing the data, enabling real-time monitoring and predictive analytics.
Analytical tools play a crucial role in predictive maintenance projects. Machine learning algorithms and statistical models can analyze historical data to identify patterns and trends that indicate potential failures. Visualization tools then present the findings in a user-friendly format, allowing maintenance teams to make informed decisions.
It is crucial to choose tools and technologies that are compatible with the existing infrastructure and capable of handling the data volume and complexity of the equipment being monitored. Additionally, organizations should consider scalability and ease of integration with other systems for future expansion.
Identifying Key Performance Indicators (KPIs)
Another vital component of a predictive maintenance project is identifying the key performance indicators (KPIs) that will be monitored and analyzed. KPIs can vary depending on the specific equipment and industry, but common examples include temperature, vibration, pressure, and energy consumption.
By defining the right KPIs, organizations can track the health and performance of their equipment accurately. This enables them to detect anomalies and deviations from normal operating conditions, which can be early indicators of potential failures.
For example, in the case of a manufacturing plant, monitoring temperature fluctuations in critical equipment can help identify overheating issues that may lead to breakdowns. Similarly, tracking vibration levels in rotating machinery can detect imbalances or misalignments that could cause significant damage if left unaddressed.
It is essential to establish baseline values for the KPIs based on historical data and equipment specifications. By comparing real-time measurements to these baselines, organizations can identify deviations and trigger maintenance actions accordingly.
Furthermore, organizations should continuously evaluate and update their KPIs as new insights and technologies emerge. This ensures that the predictive maintenance strategy remains effective and aligned with the evolving needs of the equipment and industry.
Steps to Implement a Predictive Maintenance Project
Now that we have explored the key components of a predictive maintenance project, let’s look at the necessary steps to implement it successfully.
Predictive maintenance is a proactive approach that uses data analytics and machine learning techniques to predict equipment failures before they occur. By identifying potential issues in advance, organizations can schedule maintenance activities more efficiently, reduce downtime, and optimize maintenance costs.
Planning and Designing the Project
The first step in implementing a predictive maintenance project is planning and designing the project. This involves assessing the existing maintenance processes, identifying the equipment to be monitored, and defining the project goals and objectives.
During this stage, organizations should also establish a dedicated team responsible for the project and allocate the necessary resources, including budget and personnel. The team should consist of experts from various domains, such as data science, engineering, and maintenance, to ensure a comprehensive approach.
Furthermore, organizations should consider the scalability and flexibility of the project. As the organization grows and acquires new equipment, the predictive maintenance system should be able to accommodate the changes and expand accordingly.
Data Collection and Analysis
After the planning stage, the next step is data collection and analysis. This involves installing the necessary sensors on the equipment, collecting relevant data, and storing it in a central repository for analysis.
Data collection can be done through various methods, such as IoT sensors, SCADA systems, or manual data entry. The collected data should include parameters that are indicative of equipment health, such as temperature, vibration, pressure, and electrical current.
Organizations should also establish data quality control processes to ensure the accuracy and reliability of the collected data. This may involve data cleansing, normalization, and validation techniques. Data outliers and anomalies should be identified and addressed to prevent them from affecting the accuracy of the predictive models.
Developing Predictive Models
Once the data is collected and verified, the next step is developing predictive models. This involves using advanced analytics techniques, such as machine learning algorithms, to analyze the data and generate predictive insights.
Organizations should collaborate with data scientists and domain experts to develop accurate and reliable models that can effectively predict equipment failures. The models should be trained using historical data that includes both normal and failure instances. This will enable the models to learn the patterns and indicators of potential failures.
Model performance should be continuously monitored and refined to ensure optimal accuracy. Organizations should establish a feedback loop where the models are regularly evaluated against new data and updated if necessary. This iterative process will help improve the models’ predictive capabilities over time.
Deployment and Monitoring
The final step in implementing a predictive maintenance project is deploying the predictive models and monitoring their performance. This involves integrating the models into existing systems and workflows, setting up real-time alerts and notifications, and establishing maintenance schedules based on predictive insights.
Integration with existing systems, such as enterprise asset management (EAM) or computerized maintenance management systems (CMMS), is crucial to ensure seamless communication and coordination between the predictive maintenance system and other operational processes.
Continuous monitoring of the predictive models is essential to detect any performance degradation or drift. Organizations should regularly evaluate the effectiveness of the models and make necessary adjustments to ensure optimal predictive performance. This may involve retraining the models with new data or updating the algorithms used for prediction.
Furthermore, organizations should also consider the ethical implications of predictive maintenance. Data privacy and security should be prioritized to protect sensitive information collected from the equipment. Transparency and accountability should be maintained to build trust among stakeholders.
In conclusion, implementing a predictive maintenance project involves careful planning, data collection and analysis, model development, and deployment with continuous monitoring. By following these steps, organizations can harness the power of predictive analytics to optimize maintenance activities and improve overall operational efficiency.
Overcoming Challenges in Predictive Maintenance Implementation
While the benefits of predictive maintenance are clear, implementing such a project can come with various challenges. Let’s discuss some of the common challenges and strategies to overcome them.
Dealing with Data Quality Issues
One of the significant challenges in predictive maintenance implementation is ensuring data quality. Poor-quality data can lead to inaccurate predictions and unreliable insights. Organizations should invest in data quality control processes, such as data cleansing and validation techniques, to address this challenge.
Addressing Skill Gaps
Implementing a predictive maintenance project requires specialized skills, including data scientists, domain experts, and maintenance personnel familiar with the equipment. Organizations should invest in training and development programs to bridge any skill gaps and ensure the successful implementation of the project.
Ensuring Organizational Alignment
Another challenge in implementing a predictive maintenance project is ensuring organizational alignment. This involves getting buy-in from stakeholders, including top management and maintenance teams, and aligning the project goals with the overall business objectives.
Organizations should communicate the benefits of predictive maintenance effectively and address any concerns or resistance to change. This can help create a collaborative environment and ensure the successful implementation of the project.
In conclusion, predictive maintenance is a powerful strategy that can help businesses optimize their maintenance activities and minimize downtime. Successful implementation of a predictive maintenance project requires careful planning, the right tools and technologies, and addressing various challenges along the way. By following the key components and steps outlined in this article, organizations can unlock the potential of predictive maintenance and reap its many benefits in terms of improved efficiency, reduced costs, and increased equipment reliability.